Inteligencia Artificial en Zoho Desk: Zia a tu servicio
Zia en Zoho Desk es una herramienta de inteligencia artificial desarrollada por Zoho, diseñada para mejorar la productividad de los agentes de soporte al cliente y optimizar la experiencia del cliente mediante la predicción de valores en campos clave dentro de los tickets. El objetivo de Zia es agilizar el proceso de resolución de problemas, asignar automáticamente los tickets a los agentes más apropiados, y garantizar que se brinde la atención correcta en el menor tiempo posible.
Una de las mayores dificultades en el servicio al cliente es proporcionar soluciones oportunas y adecuadas. A menudo, los agentes no cuentan con toda la información necesaria para resolver un problema por sí solos, lo que requiere que busquen aprobaciones o consulten a otros miembros del equipo. Además, los detalles incompletos o incorrectos en los tickets pueden retrasar el proceso de resolución. Para optimizar estos tiempos, es clave contar con información precisa y necesaria en los tickets desde su creación.
Zia ayuda en este proceso mediante sus capacidades predictivas. Analizando datos históricos de tickets, Zia puede predecir quién debería encargarse de un ticket, qué acción o solución se necesita, y cuánto tiempo podría llevar resolverlo. De esta forma, permite que los agentes se enfoquen en tareas más críticas, mientras Zia maneja aspectos operativos como la segmentación de tickets y su asignación automática a los agentes correctos.
Un aspecto clave es que Zia se basa en su capacidad de aprendizaje continuo, mejorando sus predicciones a medida que se le alimenta con más datos. Sin embargo, actualmente, Zia solo está disponible en inglés, aunque ya se está trabajando para incorporar soporte en otros idiomas.
El papel de Zia en la reducción del tiempo de resolución y la mejora de la satisfacción del cliente:
Los agentes de soporte tienen múltiples responsabilidades, lo que puede dificultar que ingresen cada detalle de un ticket con precisión, especialmente cuando reciben un volumen alto de solicitudes. Estos detalles son esenciales para segmentar los tickets, determinar su severidad y los costos de servicio, e implementar los acuerdos de nivel de servicio (SLA).
Las predicciones de campos de Zia pueden quitarle una gran carga de trabajo a los agentes al analizar los datos históricos y predecir los valores correctos para diversos campos. Con el tiempo, sus capacidades de aprendizaje, análisis y razonamiento se vuelven más precisas. Zia puede predecir y actualizar automáticamente los valores en campos de selección como la categoría del ticket, la prioridad y el tipo de problema, basándose en su análisis de tickets similares. También puede predecir al responsable del ticket, lo que agiliza su asignación y facilita la resolución oportuna.
Segmentación de tickets y asignación automática:
Predice los valores adecuados para campos como la severidad, el tipo de problema, el tipo de producto, el nivel de soporte y las subcategorías. Si las predicciones de Zia coinciden con el umbral de precisión configurado, se actualizarán automáticamente los valores en los campos correspondientes. Esto ahorra tiempo, garantiza que los tickets se manejen correctamente y permite una resolución rápida.
Identificación de la necesidad del cliente a partir de la descripción del ticket:
Muchas veces, los clientes levantan tickets de soporte con asuntos genéricos como “Requerimiento urgente” o “Consulta sobre transacción”, lo que requiere que los agentes lean cuidadosamente las descripciones para identificar la necesidad exacta. Este proceso puede ser tedioso y consumir mucho tiempo, especialmente cuando se manejan grandes volúmenes de tickets.
Zia puede ayudar al analizar tanto el asunto como la descripción del ticket, sugiriendo valores específicos para campos como “servicio solicitado” o “tipo de problema”. De esta manera, los agentes no tienen que pasar tanto tiempo interpretando el contenido del ticket, y el proceso de resolución se vuelve más eficiente.
Para que Zia pueda entrenarse y mejorar sus predicciones, es necesario contar con al menos 500 tickets por cada valor de campo. Las predicciones de Zia se basan en los tickets disponibles en el momento de la configuración, por lo que es importante añadir manualmente los nuevos tickets que lleguen después para que Zia pueda volver a entrenarse y ajustar sus predicciones.
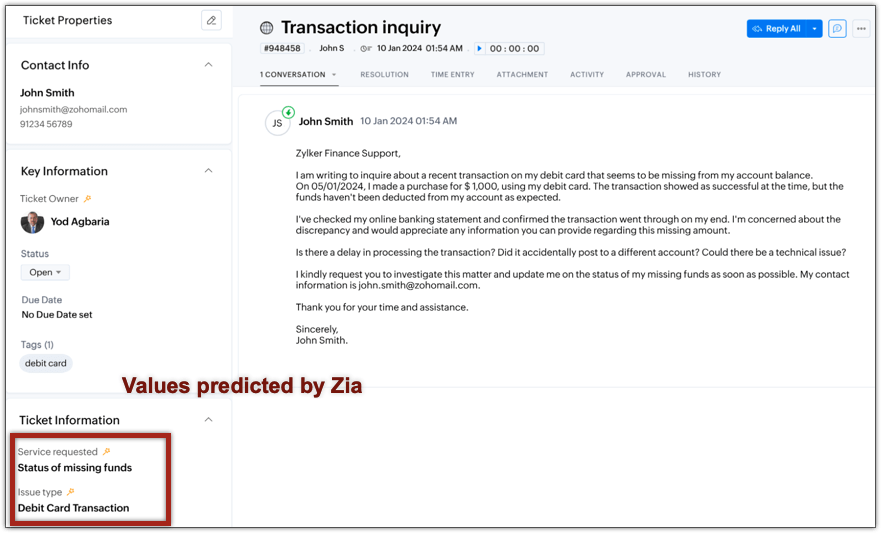
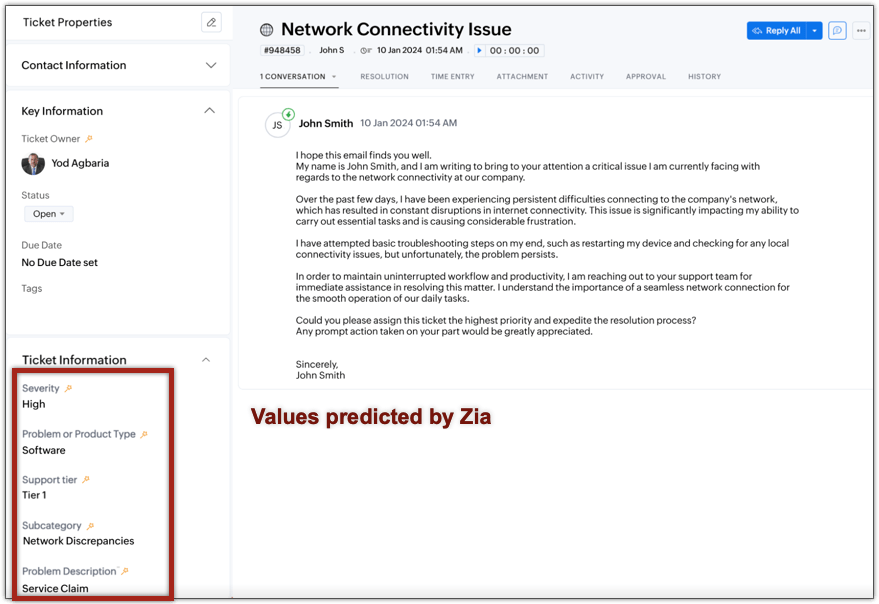
Capacitación y precisión de predicciones:
El proceso de entrenamiento de Zia implica usar los tickets existentes en la base de datos para crear patrones y predecir los valores correctos para diferentes campos. Se entrena utilizando alrededor del 80% de los tickets disponibles, y evalúa sus habilidades predictivas en el 20% restante para calcular un puntaje de precisión. Cuanto mayor sea este puntaje, más precisa será la predicción. Zia puede entrenarse con tickets específicos para ofrecer predicciones más precisas, por ejemplo, en tickets del sector tecnológico o sobre un tipo de problema particular.
Buenas prácticas para mejorar la precisión:
Para lograr un puntaje de precisión más alto, es recomendable seguir algunas mejores prácticas:
-
Usar criterios específicos para entrenar a Zia en predicciones más detalladas.
-
Proporcionar una amplia gama de datos de entrenamiento.
-
Volver a entrenar a Zia con tickets recientes.
-
Establecer un criterio de tiempo de creación para entrenar con datos específicos.
Flujo de eventos durante la predicción de campos:
Configurar predicciones de campos requiere definir algunos aspectos esenciales, como el campo que Zia debe predecir y los valores que debe analizar. Por ejemplo, si se desea que Zia prediga el tipo de problema entre opciones como “Error de código”, “Pérdida de datos” o “Corrección de bugs”, es importante que los datos de entrenamiento incluyan tickets de todas esas categorías para que la predicción sea precisa.
También es posible decidir si las predicciones se realizarán automáticamente o si los agentes deberán confirmar los valores predichos antes de actualizar el ticket. La predicción se puede ejecutar durante la creación del ticket o cuando el cliente responde a un ticket ya existente, dependiendo de las necesidades del negocio.
Importación y reentrenamiento:
A medida que se reciben nuevos tickets, es fundamental expandir el aprendizaje de Zia para garantizar que las predicciones sigan siendo precisas. Es posible importar nuevos tickets y añadirlos al sistema de predicción, lo que permite que Zia ajuste sus patrones de predicción. Las empresas también deben reentrenar a Zia periódicamente, asegurando que las predicciones se alineen con las necesidades cambiantes del negocio y los clientes.
La clave de su éxito radica en su capacidad de aprendizaje continuo, lo que garantiza que las predicciones se mantengan precisas y alineadas con las necesidades del negocio a lo largo del tiempo. Implementar Zia en la gestión de tickets es una inversión estratégica que fortalece las relaciones con los clientes y mejora la eficiencia operativa.